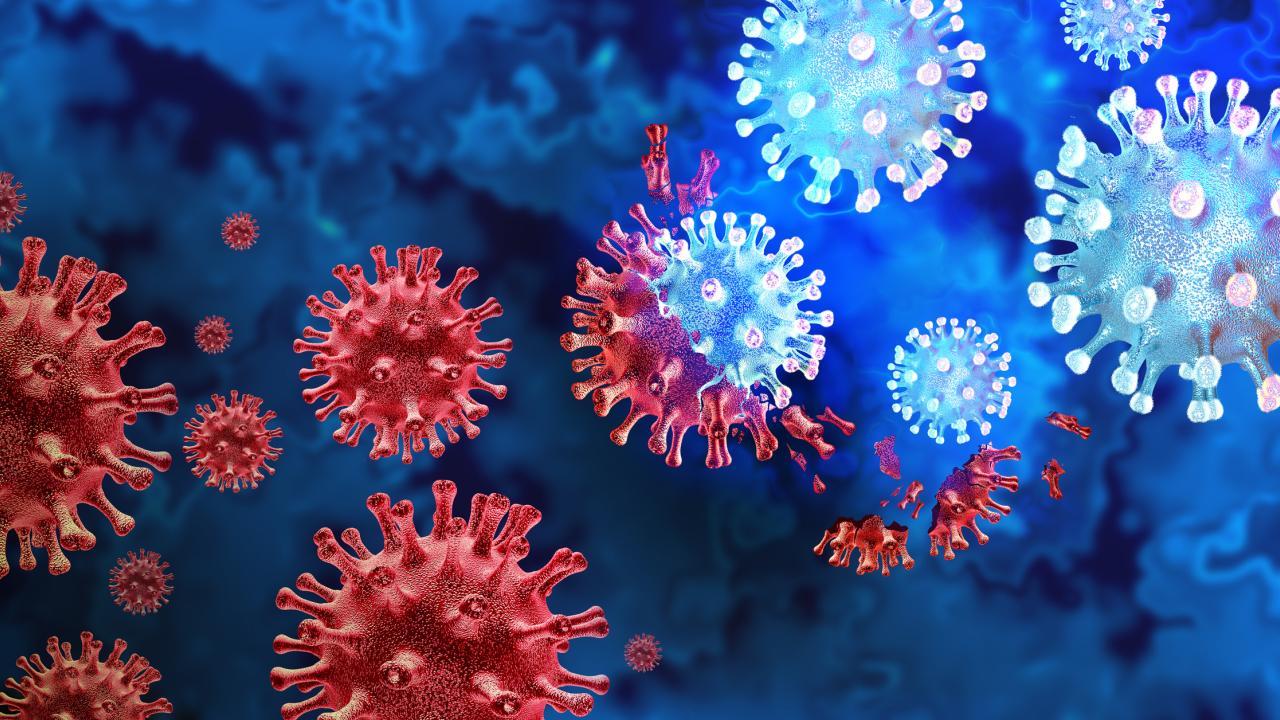
Using Machine Learning to Detect Coronavirus Threats
An artificial intelligence model has successfully identified coronaviruses capable of infecting humans, out of the thousands of viruses that circulate in wild animals. The model, developed by a team of biologists, mathematicians and physicists at the University of California, Davis, could be used in surveillance for new pandemic threats. The work was published June 8 in Scientific Reports.
Coronaviruses circulate naturally among wild animals such as bats and rodents. Occasionally, these viruses ‘spill over’ to infect humans. In some of these cases, the virus spreads to other people and may start a sustained outbreak, as with SARS in 2002 or a pandemic, such as COVID-19. If viruses with high potential to infect humans could be detected in animals before they spill over, steps could be taken to prevent or mitigate an outbreak.
“The zoonotic origins of coronaviruses and the recent pandemics show that testing of viruses from non-human hosts is a very important step in pandemic surveillance. This early surveillance could allow us to take actions and prevent viruses with human infection potential from mutating into human viruses,” said Javier Arsuaga, professor in the departments of molecular and cellular biology and of mathematics at UC Davis, and corresponding author on the paper.
Arsuaga, Professor Mariel Vazquez, departments of microbiology and molecular genetics and of mathematics, and research specialist Georgina Gonzalez-Isunza, developed a neural network model that produces a human binding potential (h-BiP) score for coronaviruses based on the ability of the virus spike protein to bind to human cells. The model was trained on data from known coronaviruses.
Identified new coronaviruses and SARS-CoV2
The model was able to identify three animal coronaviruses not previously known to bind to human cells. When the model was trained on a dataset that did not include SARS-CoV2, it successfully predicted that the SARS-CoV2 virus would bind to human receptors.
“If this software had been available in 2019, we would have predicted a strong binding of the SARS-CoV2 S protein to human cells,” Arsuaga said.
Scientists have previously tried to predict whether coronaviruses can infect humans by comparing spike protein DNA sequences. The AI approach has several advantages over this approach, Arsuaga said. It does not require any alignment of DNA sequences; it can sort spike proteins even if their sequences are quite similar; and its predictions improve as more data about spike protein binding becomes available to it. The h-BiP model can save time and effort by identifying viruses of most significance for more detailed study.
The researchers are making their software and data publicly available to the scientific community.
Additional coauthors on the paper are Professor Daniel Cox and M. Zaki Jawaid, UC Davis Department of Physics and Astronomy, and Pengyu Liu, Department of Microbiology and Molecular Genetics. The work was supported in part by a RAPID grant from the National Science Foundation.
Media Resources
- Using machine learning to detect coronaviruses potentially infectious to humans (Scientific Reports)